Comprehensive List of Researchers "Information Knowledge"
Department of Systems and Social Informatics
- Name
- KATO, Jien
- Group
- Knowledge Society and Information Systems Group
- Title
- Associate Professor
- Degree
- Dr. of Engineering
- Research Field
- computer vision / pattern recognition / machine learning and data mining
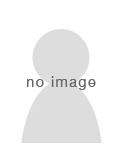
Current Research
Support for People's Social Activities by Using Image Recognition Techniques
In our research group, we conduct some fundamental research topics which create innovations. We mainly work on the following two topics, both are important and fundamental in computer vision and machine learning area.TOPICS
1. Free View Point Video Generating with Sparse Camera Setting (Keywords: stereo vision, robot vision)
Free view point video generating takes videos from multiple cameras and synthesize novel views based on the users' selection of viewpoint and direction. It is fundamental in virtual reality and plays as an essential element in three-dimensional video broadcasting/conferencing. In order to generate high quality novel videos, conventional light field rendering methods needs to capture live videos from hundreds of cameras. This makes it a severe burden for compressing and streaming these videos to the remote users, and results in such technology quite limited in usage.
In this research, we develop a novel model based rendering method towards the free view point video generating task. Our method only requires a small number of cameras. For the information lost caused by reducing the number of cameras, we additionally estimate the scene geometry, namely the dense depth map of the scene, to complement it. As wildly known, scene geometry estimation is an unsolved computer vision problem and even state-of-the-art methods could only achieve promising results under the condition that the scene is static. In our group, we explore solutions towards this problem in different ways, such like use dense descriptor as similarity measurement, introduce dynamical model to utilize the temporal smoothness, and so on. We believe, with the advance we make at the scene geometry estimation, the number of cameras that need to implement the free view point video generating will reduce to the 1/10 that need by light filed rendering method while preserving the same rendering quality.
2. Cross Domain Learning for Visual Event Recognition (Keywords: machine learning, event recognition, web mining)
Visual event recognition is an essential component in many applications such as video digest generating, visual surveillance and so on. Conventional learning based methods towards this task utilizes labeled visual data as training samples to train a set of classifiers, and apply these classifiers on novel videos to recognize events. When sufficient and strong labeled training samples are provided, these event recognition methods could achieve promising results. However, since the labeled training data are generally obtained through expensive human annotation, in case the number of labeled training samples is limited, the learned classifiers are usually not robust and do not generalize well.
As the world moves online, users distribute more and more visual data (digital images and video clips) over the internet. These visual data are usually associated with various forms of context such as captions, tags, keywords, which provide loose labels that are very valuable for learning. It opens a new door to efficiently obtain labeled visual data. However, these online visual data could not be directly used because: 1) their labels are loose and noisy; 2) their quality, size and format are ambiguous; 3) there is a gap between the web domain data and application domain data.
In this research, we propose and implement novel event recognition framework which benefit from these ever-growing amount of visual data distributed over the internet. Instead of manually providing all the labeled samples for learning, our framework leverages the visual data retrieved by web search engines and only requires a very small set of manually label ones. To deal with the issues from using web data, our work mainly focus on the following aspects: 1) we designed a course-to-fine grouping methodology which are able to eliminate the ambiguous and noise in the labels; 2) we utilize parallel processing to achieve fast normalization on the ununiformed web data; 3) we develop novel cross-domain learning technologies which could efficiently transform the learned classifiers from the web data to the one that applicable on application domain data.
Career
- Jien Kato received her Dr. of Engineering degree in information engineering from Nagoya University in 1993.
- She was an Assistant Professor at Toyama University from 1993-2000 and Academic Visitor at the University of Oxford from 1999-2000.
- In 2000, she became an Associate Professor at the Graduate School of Engineering in Nagoya University. Now, she's an Associate Professor at the Graduate School of Information Science in the same University.
Academic Societies
- Information Processing Society of Japan
- The Institute of Electronics, Information and Communication Engineers
- IEEE Computer Society
Publications
- ”HMM-based Segmentation Method for Traffic Monitoring Movies”, IEEE Transactions on Pattern Analysis and Machine Intelligence, Vol. 24, No. 9, 1291-1296 (2002).
- ”An HMM/MRF-based Stochastic Framework for Robust Vehicle Tracking”, IEEE Transactions on Intelligent Transportation Systems, Vol. 5, No. 3, 142-154 (2004).
- ”Recognition of Sound of Moving Vehicles Using Stereo Microphones”, Japan Society of Traffic Engineers, Vol. 40, No. 6, 68-79 (2005).
- ”Daily Digest Generation of Kindergartner from Surveillance Video”, IEEJ Transactions on Electronics, Information and Systems, 2010. (to appear)